On Personalisation
Content is king in gaming! What that means is, as long as it is of sufficient quality, more has always been considered better. AAA game costs increase each year (some now hitting $100,000,000+ to develop), mainly due to the increasing demand for larger and larger worlds, full of varied characters. This makes sense: the more content you produce, the more shots on target you have for engaging with the player in a meaningful way. It is clear that the recent advances in generative AI will only accelerate this, causing the previously expansive worlds to become nigh-infinite now. But is infinite content really the ultimate goal here?
Let’s look at how things are kept fresh right now. The success of series like Marvel’s “Loki” and movies like “Spiderman: Into the Spider-Verse” have shown that people love seeing new versions of familiar characters. On top of this, “Everything Everywhere All At Once” recently proved to a wider audience that the multiverse is a fantastic platform for exploring “What if…” questions. There seems to be a universal appeal for taking the familiar and looking at it from a different lens, always sitting at the boundary between known and unknown, where things are exciting, but still comprehensible.
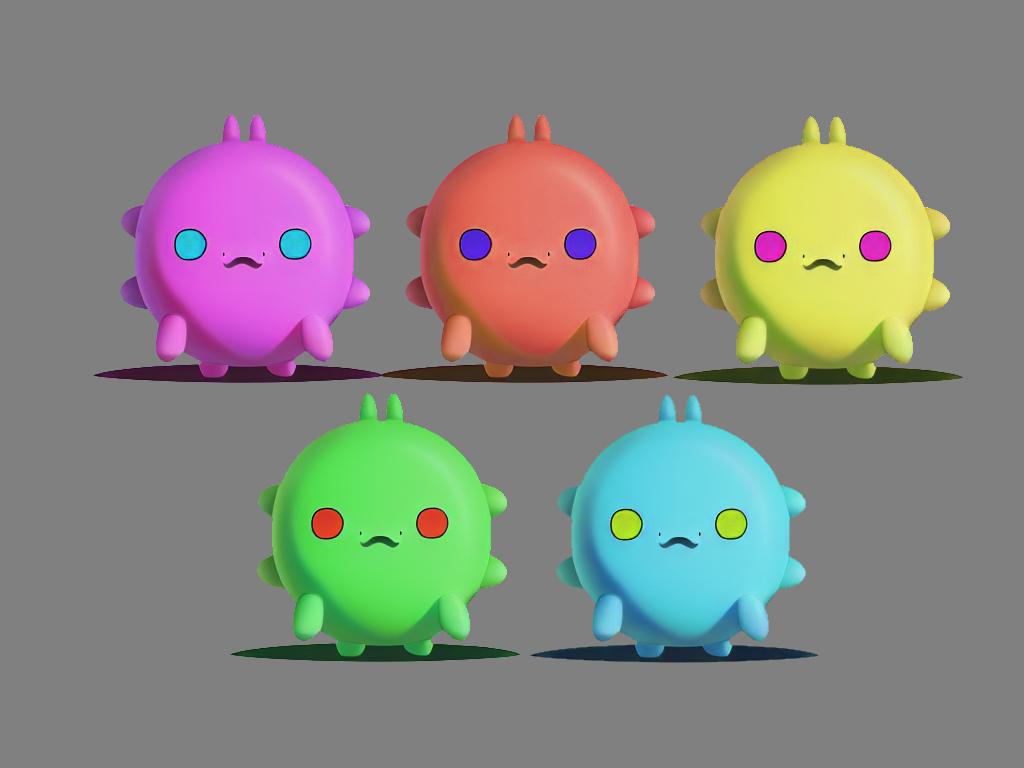
So, what is the issue with the above? The conjecture is that what we seek is actually not just “more variety”, but rather “personalisation”. The cool Noir Spiderman or the spirited anime Spidergirl out of the Spider-Verse land only for some of us, and for the rest they result in a feeling of cringe, or even worse, a reason to disconnect from the experience. As technology allows us to generate more and more variants of new and familiar concepts, audiences need help navigating this landscape without feeling overwhelmed. But how to do that?
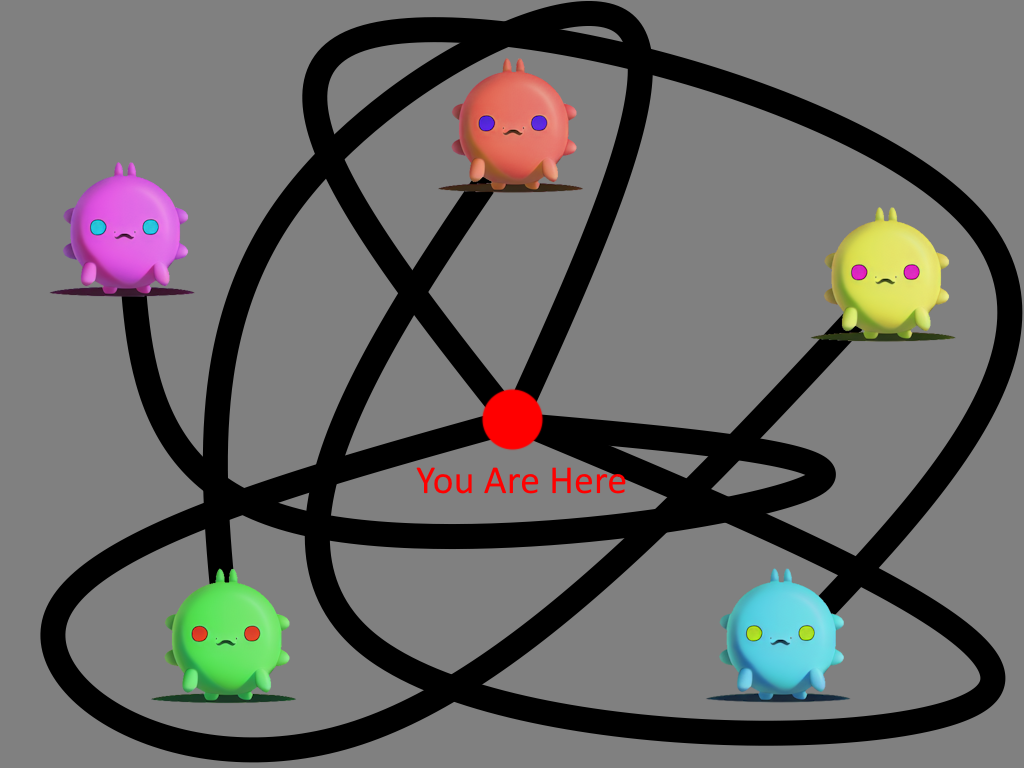
It all starts with knowing the audience. Luckily, recommendation engines completely transformed the entire commercial sector by being able to pick out people’s preferences based on secondary cues, such as what they engage with and what they ignore. This is convenient, as, if you expect people to spend the time to describe what they want exactly, you’re in for a bad time.
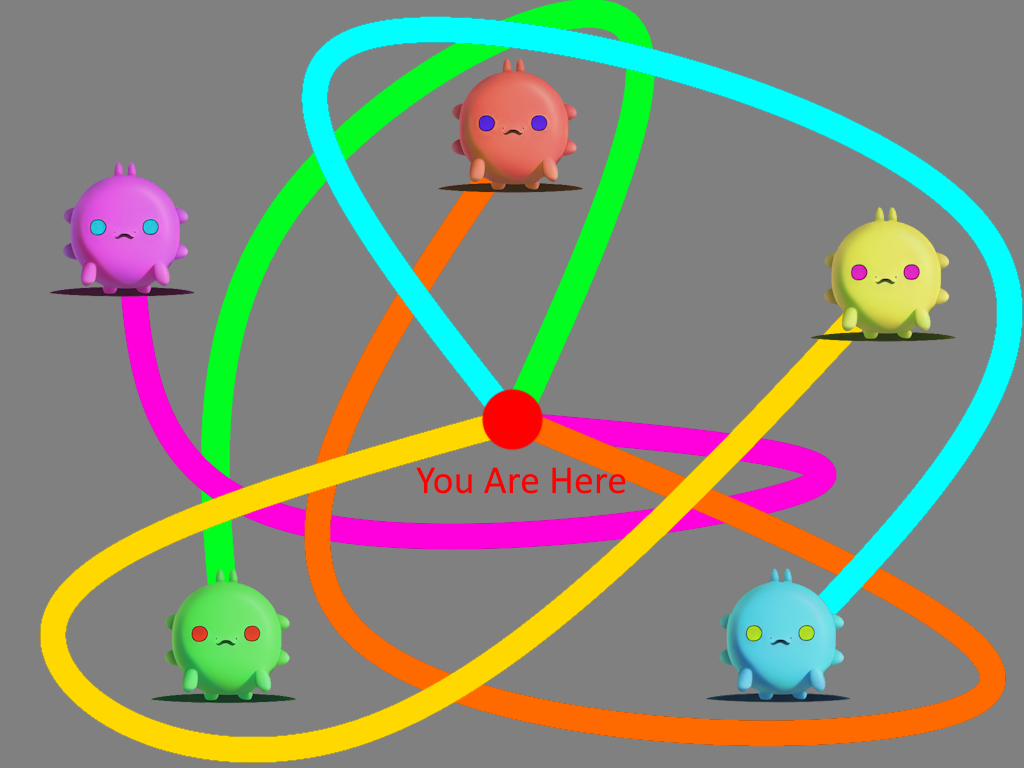
So we just use a recommendation engine and we’re good, right? We can actually do better. We’re now sitting on top of technology which has the potential to not only find the closest pre-existing match, but actually generate something directly addressing a certain preference. What’s more, this requires input on the part of the person interacting with the content and luckily, in gaming, we have that for free! This interactivity makes the difference between passively observing the answers to the “What If…” questions of others, and being able to ask your own, e.g. as seen (in a limited capacity) in “The Stanley Parable”.
Once you have...
At MLC we’re interested in studying the problems related to personalisation in interactive experiences, namely:
-
How novel personalisation technologies can be meaningfully integrated into gaming
-
How to obtain vital personalisation information from players in an immersive and non-disruptive manner
-
How gaming experiences can be augmented live, while being played, and past the original conceptions of the game’s designers
-
How to keep providing players with exciting, but comprehensible challenges
If these sound like things you’d like to work on, have a look at our jobs page or get in touch directly by sending a message to hello@martianlawyers.club
Content is king in gaming! What that means is, as long as it is of sufficient quality, more has always been considered better. AAA game costs increase each year (some now hitting $100,000,000+ to develop), mainly due to the increasing demand for larger and larger worlds, full of varied characters. This makes sense: the more content you produce, the more shots on target you have for engaging with the player in a meaningful way. It is clear that the recent advances in generative AI will only accelerate this, causing the previously expansive worlds to become nigh-infinite now. But is infinite content really the ultimate goal here?
Let’s look at how things are kept fresh right now. The success of series like Marvel’s “Loki” and movies like “Spiderman: Into the Spider-Verse” have shown that people love seeing new versions of familiar characters. On top of this, “Everything Everywhere All At Once” recently proved to a wider audience that the multiverse is a fantastic platform for exploring “What if…” questions. There seems to be a universal appeal for taking the familiar and looking at it from a different lens, always sitting at the boundary between known and unknown, where things are exciting, but still comprehensible.
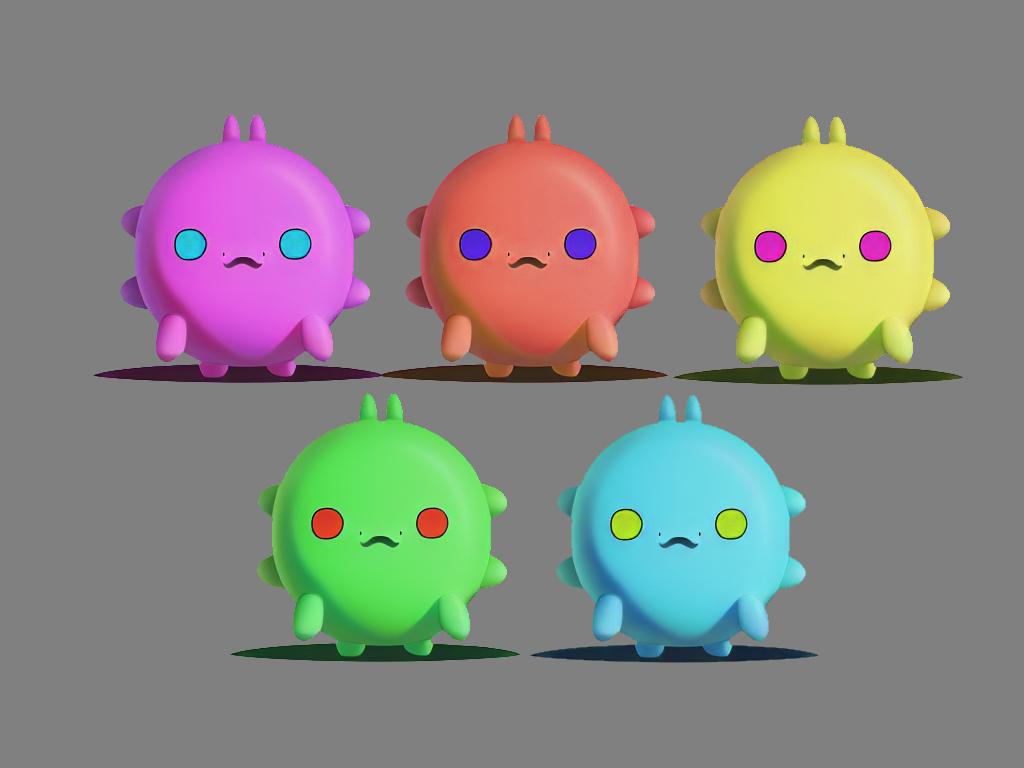
So, what is the issue with the above? The conjecture is that what we seek is actually not just “more variety”, but rather “personalisation”. The cool Noir Spiderman or the spirited anime Spidergirl out of the Spider-Verse land only for some of us, and for the rest they result in a feeling of cringe, or even worse, a reason to disconnect from the experience. As technology allows us to generate more and more variants of new and familiar concepts, audiences need help navigating this landscape without feeling overwhelmed. But how to do that?
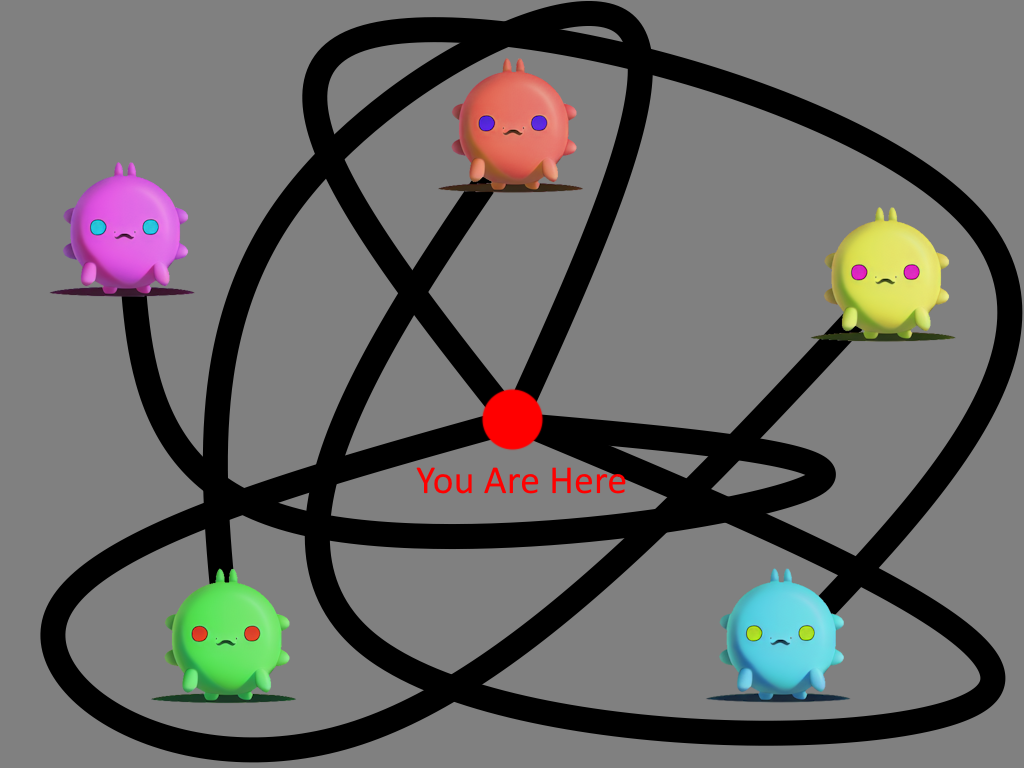
It all starts with knowing the audience. Luckily, recommendation engines completely transformed the entire commercial sector by being able to pick out people’s preferences based on secondary cues, such as what they engage with and what they ignore. This is convenient, as, if you expect people to spend the time to describe what they want exactly, you’re in for a bad time.
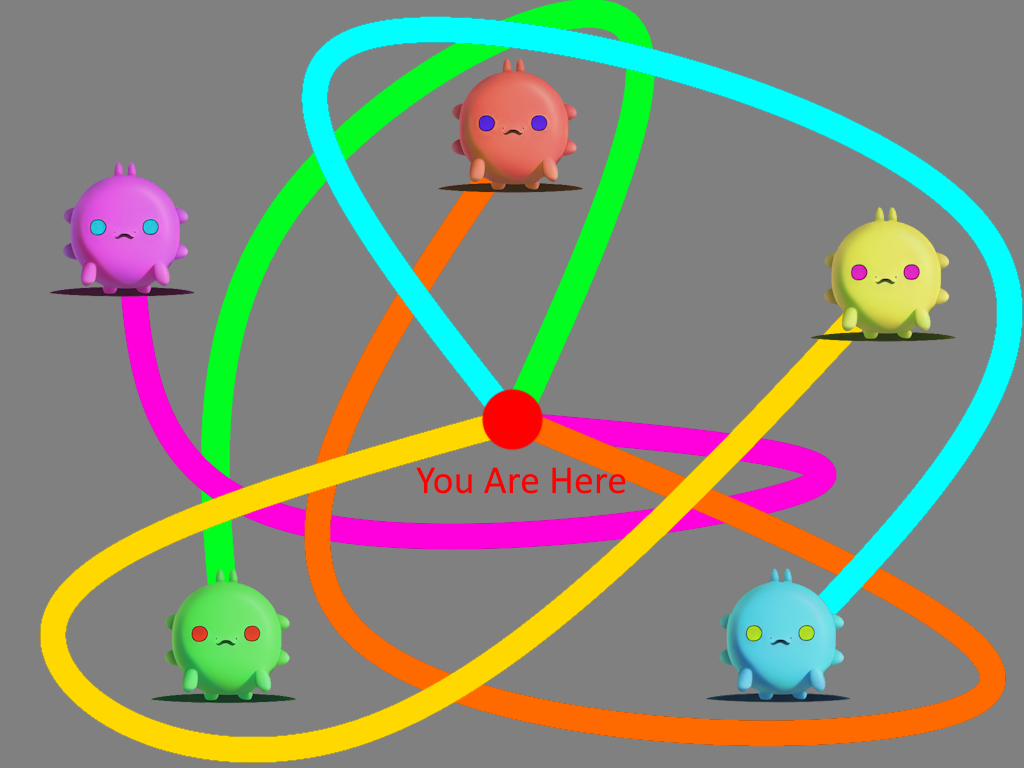
So we just use a recommendation engine and we’re good, right? We can actually do better. We’re now sitting on top of technology which has the potential to not only find the closest pre-existing match, but actually generate something directly addressing a certain preference. What’s more, this requires input on the part of the person interacting with the content and luckily, in gaming, we have that for free! This interactivity makes the difference between passively observing the answers to the “What If…” questions of others, and being able to ask your own, e.g. as seen (in a limited capacity) in “The Stanley Parable”.
Once you have...
At MLC we’re interested in studying the problems related to personalisation in interactive experiences, namely:
-
How novel personalisation technologies can be meaningfully integrated into gaming
-
How to obtain vital personalisation information from players in an immersive and non-disruptive manner
-
How gaming experiences can be augmented live, while being played, and past the original conceptions of the game’s designers
-
How to keep providing players with exciting, but comprehensible challenges
If these sound like things you’d like to work on, have a look at our jobs page or get in touch directly by sending a message to hello@martianlawyers.club